Beating the Odds: When Teams Outperform Their Projections
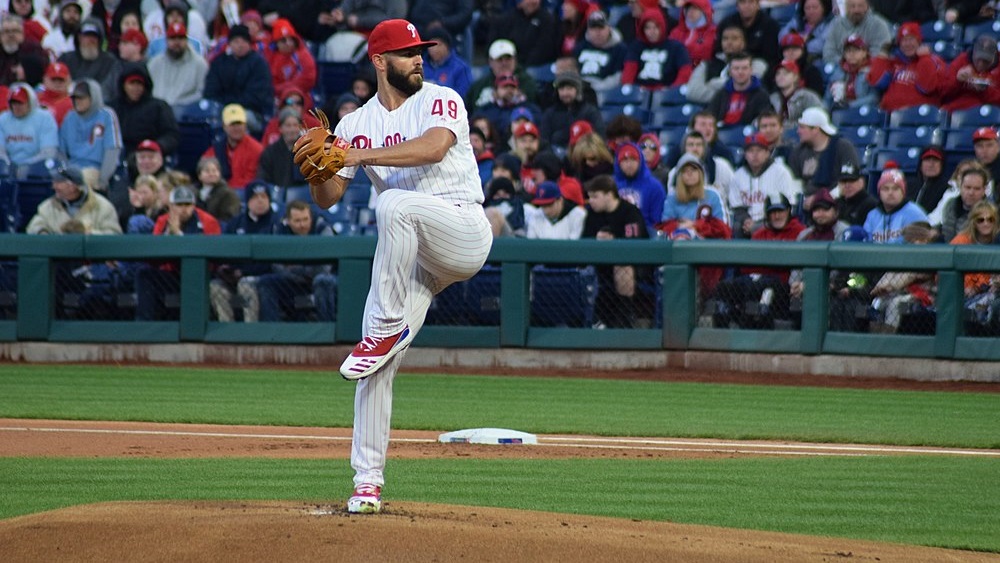
The Phillies have under-performed their projections the most this season. (via Ian D’Andrea)
Projection systems have a purpose in baseball. They’re the most measured form of expectation heading into a season. They set a baseline for how good or bad a player or team will be, simultaneously pointing out possible strengths and weaknesses.
Predicting performance in baseball isn’t a perfect science. It isn’t meant to be. The process is tailored to accuracy. This involves a variety of methods, including adjustments brought on by changing factors of performance, regression to the mean, and accounting for age. What projections don’t account for are the actual changes a player made. Maybe a player adjusted his swing, molding him into an entirely different hitter than he was before (i.e. Max Muncy). Maybe a pitcher made a change to his repertoire or release point, making a lot of the previous data on him irrelevant (i.e. Lucas Giolito).
Players like these perhaps make up the hardest part of projecting performance, for a variety of reasons. A lot of times, their changes go unnoticed before the season. Most of the time, there is a low level of reliability in the thought of these changes showing up in actual results.
Thinking deeper, these players that blew past their projections can still be a contributing factor into the analysis of player development. For example, heading into the 2017 season, Cardinals outfielder Jose Martinez was projected to put up a .311 wOBA (per Steamer), below the major league average, and with his lackluster defensive skills, making him appear to be a below-replacement-level hitter. But while spending his age-26 and age-27 seasons with the Royals Triple-A affiliate, the Cardinals noticed something that suggested he could perform at a high level in the majors.
Cardinals officials first eyed Martinez a year earlier, when he was swinging his way to a Pacific Coast League batting crown for Kansas City’s Triple-A affiliate in Omaha. He hit .384/.461/.563 that season, his 12th in pro ball without a big-league taste. The breakout year failed to make a major prospect out of Martinez, who by that point was 26, had cycled through three organizations, undergone three knee surgeries and never hit for power. Back home in Venezuela that winter, he wondered what he still had to prove.”
After a few adjustments, Martinez went on to post a .379 wOBA at the major league level for the 2017 season, ranking in the top eight percent of major league hitters and making him one of the biggest over-performers relative to his preseason projections that season.
So who deserves the credit for Martinez’s surprising season? Obviously Martinez himself gets a ton of credit for executing the changes necessary for him to perform, but one would think a fair amount of recognition should go to the player development and scouting staff for the Cardinals for the assistance in making his impressive season possible.
Measuring the skills of an organization’s player development system is tricky. Sure, the ones that are good at what they do are well known, while some of the bad ones may be pointed out from time to time. But everything in between lurks in the unknown. Quantifying the results in this area is tough, but a method might lie in preseason projections.
One of the more prominent projection systems out there is the well-known Steamer projections. They have been putting out preseason projections for both hitters and pitchers dating all the way back to the 2010 season. The accuracy of their method is strong and through recent history has only been getting better.
For my analysis, I’ll be using preseason wOBA projections for hitters and SIERA projections for pitchers and comparing their projections to their in-season results in those respective metrics, all data coming from Steamer. To keep recency into play, I’ll only be going back to the 2015 season, hopefully giving a good view of the current landscape of baseball while keeping the sample size ideal.
The goal here will be to determine the level at which teams over-perform their preseason projections on a player-by-player basis. The thought heading into this investigation would be that teams with good player development systems would over-perform their preseason projections more often, while teams with bad ones would have their players under-perform their projections more often.
First, to set the basis, here are the hitters with at least 200 plate appearances in a season dating back to 2015 who have over-performed relative to their projections the most.
Name | Season | Team | Age | Projected PA | Projected wOBA | Actual wOBA | wOBA Differential |
---|---|---|---|---|---|---|---|
Juan Soto | 2018 | WAS | 19 | 1 | 0.266 | 0.392 | 0.125 |
Yordan Alvarez | 2019 | HOU | 22 | 1 | 0.311 | 0.436 | 0.125 |
Max Muncy | 2018 | LAN | 27 | 1 | 0.288 | 0.407 | 0.119 |
Gary Sanchez | 2016 | NYA | 23 | 85 | 0.313 | 0.425 | 0.112 |
Matt Olson | 2017 | OAK | 23 | 64 | 0.300 | 0.411 | 0.111 |
Andres Blanco | 2015 | PHI | 31 | 66 | 0.266 | 0.372 | 0.106 |
Carlos Correa | 2015 | HOU | 20 | 1 | 0.261 | 0.365 | 0.105 |
Fernando Tatis Jr. | 2019 | SDN | 20 | 336 | 0.295 | 0.398 | 0.103 |
Ryan Raburn | 2015 | CLE | 34 | 157 | 0.294 | 0.397 | 0.103 |
Aaron Judge | 2017 | NYA | 25 | 392 | 0.331 | 0.430 | 0.099 |
Jeff McNeil | 2018 | NYN | 26 | 1 | 0.271 | 0.368 | 0.097 |
Zack Cozart | 2017 | CIN | 31 | 483 | 0.296 | 0.392 | 0.096 |
Sandy Leon | 2016 | BOS | 27 | 1 | 0.267 | 0.362 | 0.095 |
Rhys Hoskins | 2017 | PHI | 24 | 1 | 0.322 | 0.417 | 0.095 |
Alex Bregman | 2016 | HOU | 22 | 1 | 0.242 | 0.336 | 0.093 |
Bryce Harper | 2015 | WAS | 22 | 560 | 0.370 | 0.461 | 0.091 |
Bryan Reynolds | 2019 | PIT | 24 | 7 | 0.304 | 0.392 | 0.088 |
Keston Hiura | 2019 | MIL | 22 | 20 | 0.302 | 0.389 | 0.087 |
Marwin Gonzalez | 2017 | HOU | 28 | 276 | 0.295 | 0.382 | 0.087 |
Kyle Schwarber | 2015 | CHN | 22 | 1 | 0.279 | 0.364 | 0.085 |
A noticeable trend among these 20 players is their respective ages. Thirteen of them are 25 or younger, suggesting that over-performance in projections can be most common in players with little to no data in the majors. There are a few notable breakouts here, such as Max Muncy, Marwin Gonzalez, and Zack Cozart, while a couple of them ran BABIP-fueled campaigns to success, like Sandy Leon, Andres Blanco, and Ryan Raburn.
Looking to the opposite end of the spectrum, here are the 20 hitters who under-performed their projections the most.
Name | Season | Team | Age | Projected PA | Projected wOBA | Actual wOBA | wOBA Differential |
---|---|---|---|---|---|---|---|
Chris Davis | 2018 | BAL | 32 | 524 | 0.342 | 0.239 | -0.103 |
Travis Shaw | 2019 | MIL | 29 | 598 | 0.338 | 0.246 | -0.092 |
Jung Ho Kang | 2019 | PIT | 32 | 428 | 0.347 | 0.255 | -0.091 |
Eduardo Nunez | 2019 | BOS | 32 | 238 | 0.320 | 0.233 | -0.086 |
Garrett Hampson | 2019 | COL | 24 | 348 | 0.334 | 0.248 | -0.086 |
Prince Fielder | 2016 | TEX | 32 | 635 | 0.361 | 0.276 | -0.084 |
Victor Martinez | 2015 | DET | 36 | 555 | 0.370 | 0.286 | -0.084 |
Yan Gomes | 2016 | CLE | 28 | 434 | 0.308 | 0.225 | -0.083 |
Dexter Fowler | 2018 | SLN | 32 | 555 | 0.343 | 0.260 | -0.082 |
Lewis Brinson | 2019 | MIA | 25 | 497 | 0.293 | 0.211 | -0.082 |
Ramon Flores | 2016 | MIL | 24 | 199 | 0.330 | 0.250 | -0.080 |
Miguel Cabrera | 2017 | DET | 34 | 621 | 0.391 | 0.313 | -0.079 |
Jeff Mathis | 2019 | TEX | 36 | 275 | 0.271 | 0.193 | -0.078 |
Rene Rivera | 2015 | TBA | 31 | 386 | 0.287 | 0.215 | -0.072 |
Jose Bautista | 2017 | TOR | 36 | 540 | 0.366 | 0.295 | -0.072 |
Tyler Saladino | 2017 | CHA | 27 | 272 | 0.294 | 0.223 | -0.072 |
Hunter Pence | 2018 | SFN | 35 | 493 | 0.325 | 0.254 | -0.071 |
Roberto Perez | 2018 | CLE | 29 | 218 | 0.305 | 0.236 | -0.069 |
Lewis Brinson | 2018 | MIA | 24 | 398 | 0.317 | 0.248 | -0.069 |
Michael Saunders | 2017 | PHI | 30 | 483 | 0.324 | 0.256 | -0.068 |
The most common trend among these hitters once again is age, as 11 of the 20 hitters were over the age of 30. Hitters like Chris Davis, Prince Fielder, Miguel Cabrera, and Jose Bautista followed up season(s) of high-level offensive production with plummeting performances when the aging curve hit them. Then there are prospects like Lewis Brinson and Garrett Hampson, who couldn’t reach their expectations once arriving in the big leagues.
With the biggest over-performances in SIERA among pitchers since 2015, it’s mostly relievers. You would have to get by 59 relievers in that time to find the biggest over-performance by a starting pitcher, that being 2019 Lucas Giolito. Pitching in smaller sample sizes, relievers can be really volatile and hard to project. There is no clear distinction with this group, as most of them are just pitchers who put up really good seasons.
Name | Season | Team | Age | Projected SIERA | Actual SIERA | SIERA Difference |
---|---|---|---|---|---|---|
Edwin Diaz | 2016 | SEA | 22 | 4.34 | 1.82 | -2.52 |
Seranthony Dominguez | 2018 | PHI | 23 | 4.96 | 2.81 | -2.15 |
Caleb Ferguson | 2018 | LAN | 21 | 4.79 | 2.82 | -1.97 |
Michael Lorenzen | 2016 | CIN | 24 | 4.62 | 2.75 | -1.87 |
Chad Green | 2017 | NYA | 26 | 3.78 | 2.03 | -1.75 |
Tommy Kahnle | 2017 | CHA | 27 | 3.92 | 2.25 | -1.67 |
Roberto Osuna | 2015 | TOR | 20 | 4.46 | 2.81 | -1.65 |
Jose Leclerc | 2018 | TEX | 24 | 4.25 | 2.60 | -1.65 |
Craig Kimbrel | 2017 | BOS | 29 | 2.81 | 1.18 | -1.63 |
Carson Smith | 2015 | SEA | 25 | 3.67 | 2.04 | -1.63 |
Daniel Coulombe | 2016 | OAK | 26 | 4.46 | 2.83 | -1.63 |
Tanner Scott | 2018 | BAL | 23 | 4.79 | 3.18 | -1.61 |
Taylor Rogers | 2016 | MIN | 25 | 4.64 | 3.12 | -1.52 |
Mark Lowe | 2015 | CLE | 32 | 4.02 | 2.58 | -1.44 |
Josh Hader | 2018 | MIL | 24 | 3.13 | 1.70 | -1.43 |
Joe Smith | 2017 | TOR | 33 | 3.74 | 2.33 | -1.41 |
Sam Dyson | 2015 | MIA | 27 | 3.78 | 2.40 | -1.38 |
Ryan Madson | 2017 | OAK | 36 | 3.69 | 2.32 | -1.37 |
Jace Fry | 2018 | CHA | 24 | 4.20 | 2.84 | -1.36 |
Keone Kela | 2015 | TEX | 22 | 4.06 | 2.70 | -1.36 |
For the most under-performing pitchers, it’s a bit of a mixed bag. In terms of age, it’s a pretty even distribution. This array of players probably speaks to volatility also, with most of these pitchers being projected at the least to have acceptable seasons before proceeding to fall apart for one reason or another.
Name | Season | Team | Age | Projected SIERA | Actual SIERA | SIERA Difference |
---|---|---|---|---|---|---|
Jeff Samardzija | 2018 | SFN | 33 | 3.51 | 5.96 | 2.45 |
Tyson Ross | 2017 | TEX | 30 | 3.85 | 6.17 | 2.32 |
Blake Treinen | 2019 | OAK | 31 | 2.74 | 5.04 | 2.30 |
Jeurys Familia | 2019 | NYN | 29 | 3.03 | 5.31 | 2.28 |
Tyler Chatwood | 2018 | CHN | 28 | 4.07 | 6.28 | 2.21 |
Drew Hutchison | 2018 | PHI | 27 | 3.33 | 5.51 | 2.18 |
Tayron Guerrero | 2019 | MIA | 28 | 3.61 | 5.77 | 2.16 |
Manny Banuelos | 2019 | CHA | 28 | 3.72 | 5.86 | 2.14 |
Shelby Miller | 2019 | TEX | 28 | 4.26 | 6.28 | 2.02 |
Odrisamer Despaigne | 2017 | MIA | 30 | 3.78 | 5.72 | 1.94 |
Matt Harvey | 2017 | NYN | 28 | 3.54 | 5.44 | 1.90 |
Chi Chi Gonzalez | 2019 | COL | 27 | 4.56 | 6.45 | 1.89 |
Bryan Mitchell | 2018 | SDN | 27 | 4.00 | 5.89 | 1.89 |
Erick Fedde | 2019 | WAS | 26 | 3.82 | 5.63 | 1.81 |
Tyler Kinley | 2019 | MIA | 28 | 3.80 | 5.59 | 1.79 |
Wandy Peralta | 2018 | CIN | 26 | 3.70 | 5.43 | 1.73 |
Dan Straily | 2019 | MIA | 30 | 4.38 | 6.08 | 1.70 |
Tyler Glasnow | 2017 | PIT | 23 | 3.93 | 5.62 | 1.69 |
Tony Cingrani | 2016 | CIN | 26 | 3.33 | 5.01 | 1.68 |
Neftali Feliz | 2017 | MIL | 29 | 3.31 | 4.97 | 1.66 |
Piecing all of this together can give a good understanding of what teams have their players over- or under-perform their projections the most, ideally giving a useful measurement of the success of an organization’s player development staff. Initially, the plan to compile this data simply was going to be to find the average of each team’s difference in projections and results, separated by hitters and pitchers. That plan was foiled though, as it didn’t take long to realize the consistency of league-wide differences between projections and results varied year to year to a strong extent, likely caused by the league-wide offensive variation resulting from changes to the makeup of the baseball.
Season | Average wOBA Difference | Average SIERA Difference |
---|---|---|
2015 | 0.005 | -0.07 |
2016 | 0.009 | 0.20 |
2017 | 0.010 | 0.37 |
2018 | -0.003 | 0.12 |
2019 | 0.005 | 0.55 |
An adjustment was needed for this effect, so I found the z-score of the projections-results difference for each player compared to their respective season, ultimately converting their differences into a plus stat.
Now after adding context to the data and finding the average differences in wOBA and SIERA for each team, there is a final calculation.
Team | Average wOBA Difference |
---|---|
CIN | 110 |
TBA | 108 |
SLN | 107 |
ATL | 106 |
LAN | 106 |
TOR | 105 |
HOU | 104 |
PIT | 104 |
MIL | 103 |
NYN | 103 |
MIA | 102 |
CHN | 102 |
ARI | 99 |
DET | 99 |
BOS | 99 |
TEX | 99 |
OAK | 99 |
NYA | 98 |
KCA | 98 |
PHI | 98 |
CLE | 98 |
COL | 97 |
SDN | 97 |
SEA | 97 |
SFN | 96 |
CHA | 95 |
WAS | 94 |
MIN | 93 |
LAA | 91 |
BAL | 89 |
Team | Average Difference SIERA z-score |
---|---|
HOU | 115 |
CLE | 110 |
NYA | 108 |
MIN | 106 |
LAA | 105 |
OAK | 104 |
BOS | 104 |
TOR | 104 |
SEA | 103 |
BAL | 103 |
TBA | 103 |
LAN | 102 |
KCA | 102 |
DET | 102 |
TEX | 102 |
CHA | 100 |
SDN | 100 |
ARI | 99 |
SLN | 99 |
WAS | 97 |
MIL | 97 |
ATL | 97 |
PIT | 95 |
NYN | 94 |
SFN | 94 |
MIA | 94 |
COL | 91 |
CIN | 91 |
CHN | 91 |
PHI | 91 |
Averaging these two tables gives the final product of an attempt to measure the success level of a player development staff.
Team | Average wOBA Difference z-score | Average Difference SIERA z-score | Average z-score |
---|---|---|---|
HOU | 104 | 115 | 109 |
TBA | 108 | 103 | 105 |
TOR | 105 | 104 | 104 |
LAN | 106 | 102 | 104 |
CLE | 98 | 110 | 104 |
NYA | 98 | 108 | 103 |
SLN | 107 | 99 | 103 |
ATL | 106 | 97 | 101 |
BOS | 99 | 104 | 101 |
OAK | 99 | 104 | 101 |
CIN | 110 | 91 | 101 |
DET | 99 | 102 | 100 |
MIL | 103 | 97 | 100 |
TEX | 99 | 102 | 100 |
SEA | 97 | 103 | 100 |
KCA | 98 | 102 | 100 |
MIN | 93 | 106 | 100 |
PIT | 104 | 95 | 99 |
ARI | 99 | 99 | 99 |
NYN | 103 | 94 | 99 |
SDN | 97 | 100 | 98 |
LAA | 91 | 105 | 98 |
MIA | 102 | 94 | 98 |
CHA | 95 | 100 | 98 |
CHN | 102 | 91 | 96 |
BAL | 89 | 103 | 96 |
WAS | 94 | 97 | 95 |
SFN | 96 | 94 | 95 |
COL | 97 | 91 | 94 |
PHI | 98 | 91 | 94 |
Could the top team have been any less surprising? The Astros, well known for their success in scouting and player development, have had their players outperform their projections more than any other organization’s players over the last five seasons, with instances like Gerrit Cole, Wade Miley, Dallas Keuchel, Yordan Alvarez, and Marwin Gonzalez leading the way, among many others. Other organizations ranking towards the top are familiar when the topic turns to good player development systems, including the Rays, Dodgers, Indians, and Yankees.
Occupying spots at the bottom are the White Sox, Cubs, Orioles, Rockies, and Phillies. It is worth mentioning that the Orioles, the fifth most under-performing team in terms of projections, have outperformed both their wOBA and SIERA projections more this season than any of the last four. In 2019, only eight teams have had their hitters out-perform their wOBA projections more, and only seven teams have had their pitchers out-perform their SIERA projections more. This comes right after the hiring of Mike Elias and Sig Mejdal, two key members of the Astros brain trust during their rebuild and success, to help replace the former front-office regime.
The big question is, how can this information be utilized going forward? To answer, there is recent reasonable evidence to suggest this data can be factored into future projections. This capability lies in the fact that performance relative to projections can be predictive on a team basis, showing a low level of correlation.
Metric | Team Performance YoY Correlation (R=) |
---|---|
wOBA | 0.164 |
SIERA | 0.203 |
The idea behind factoring these numbers into future projections would be operating under the thought that a team’s player development system has an impact on how a single player performs. This obviously isn’t the case 100 percent of the time, but if it can create more predictiveness in projections, it’s useful. By using team projection performance data from the past two seasons (more recency causes more reliability) and adding the factor into a player’s projections (using Steamer again), it is shown there is indeed an increase in correlation with projections and results for both hitters and pitchers. (The sample here is all hitters with at least 200 plate appearances and pitchers with at least 40 innings pitched in 2019.)
Metric | Sample (n=) | Initial Projection vs Results Correlation (R=) | Projections Weighted w/ Team Performance vs Results Correlations (R=) |
---|---|---|---|
wOBA | 348 | 0.522 | 0.572 |
SIERA | 355 | 0.630 | 0.669 |
Factoring an organization’s track-record of player development through its performance relative to projections into a single player’s projections never came to mind. Once it did, the idea of doing so initially appeared dubious to me, mostly due to an array of unknown inputs.
But what flipped my thoughts on this was the discovery of some level of year-to-year correlation of team performance against their projections. With consistency existing, it wasn’t crazy to think this data could be used as another input into a players’ projection.
There are still some issues with how projection vs. result can be used to measure a player development system’s success. The main concern is how to deal with front offices that have had recent turnover in this department. But for teams with longevity in their track records, there definitely is usefulness in this practice.
Player development is becoming more and more of a mainstay in this current age of baseball as technology and data collecting continue to grow. With top teams like the Astros, Dodgers, Rays, Yankees, and Indians continuing to fill their rosters with players who exceed expectations, it seems increasingly important to have data to measure and predict with.
References & Resources
- Credit to Kyle Boddy, for giving me this idea through a tweet of his
- This obviously couldn’t have been done without the extensive work Steamer Projections does and the past projection data they have on their website
- FanGraphs Splits Leaderboard for results data
Impressive data, though this effect seems likely to be at least partially explained by inaccurate park/league factors in the minors.
The correl. of wOBA and SIERA z-scores is -0.19. I.e., teams that have hitter out-performance tend to have pitcher under-performance. If the effect was due to overall player development skill, one would expect a small, positive correlation between the two.
P.S. There’s a typo in the tables listing Phi twice.
Seems as tho there are multiple PHI and no PIT, so I can’t see how poorly the Pirates have done.
I’m a little late to the discussion, but you are missing PIT but have PHI twice in the charts.