KATOH Goes to College: Projecting College Pitchers
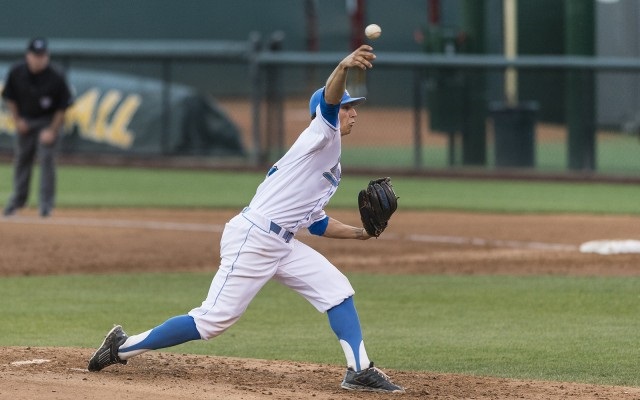
James Kaprielian projects to have the best WAR of any college pitcher available. (via Daily Bruin)
On Friday, I gave a rundown of the KATOH model I built to forecast college hitters’ major league performance. Then, I applyied my work to some of the hitters who figure to be taken in the early portion of tonight’s draft. Today, I’m back with more KATOH. This time, I’ll run through my efforts to project college pitchers, using exclusively their college statistics.
The framework of my methodology looks a lot it did for the model I used to forecast hitters. Again, I calculated each player’s regressed, conference-adjusted stats. Then, I deployed a series of probit regressions to see what stuck when it came to forecasting major league performance. These are the thresholds I chose to use in my analysis, regarding a hitter’s performance through age 28:
- Playing in the majors (at least one game)
- >1 WAR
- >2 WAR
- >3 WAR
- >4 WAR
- >5 WAR
- >6 WAR
- >7 WAR
- >8 WAR
- >9 WAR
- >10 WAR
To account for a pitcher’s level of competition, I included his conference as a categorical variable in my regression models. My source for these college stats — Chris Long’s database — had data going back only to 2002, and it’s too soon to do much with the last few years of college data. The jury’s still out on a player who’s in his mid-20s or younger. That left me with only a few years of college data to play with. Due to these data constraints, I was forced to exclude the conferences that have produced only a handful of big leaguers over the time period I examined. Below are the conferences I included, ranked from highest to lowest using the coefficients my regression model spat out for said conferences in my “Making the Majors” model.
- Pac 12 Conference
- Big West Conference
- Conference USA
- Big 12 Conference
- Atlantic Coast Conference (ACC)
- Southeastern Conference (SEC)
- Mountain West Conference
- Big South Conference
- Missouri Valley Conference
- American Athletic Conference (AAC)
- Big 10 (B1G) Conference
- Atlantic Sun Conference
- Colonial Athletic Association (CAA)
These data don’t say that the Pac-12 conference is the best college baseball conference. In fact, this is answering a different question entirely. All this says is that given a player with identical numbers from every conference, the one coming from the Pac-12 has the best shot at playing in the majors.
Unfortunately, Chris Long’s pitching database did not include batters faced for its pitchers. This meant that I couldn’t use per-batter rate stats — like strikeout rate and walk rate — in my analysis. Instead, I had to settle for per-inning metrics, such as strikeouts or walks per inning pitched. While less than ideal, I don’t think this is too big of an impediment. Per-inning metrics generally correlate pretty will with per-batter metrics, and should act as a good-enough proxy in this case. When it was all said and done, these were the inputs I settled on for my models. The variables related to a pitcher’s performance were regressed to league average, and were centered based on the average for that pitcher’s conference.
KATOH College Model Variables |
---|
Variable | Definition |
BB% | BB/IP |
K% | K/IP |
H% | H/IP |
GS% | Games Started / Total Appearances |
Conference | Pitcher’s team’s athletic conference |
Class year | Hitter’s listed class year |
The glaring omission here is home run rate, which is obviously a big part of a pitcher’s game. As DIPS theory has taught us, home runs are one of the few things over which a pitcher has a meaningful amount of control, along with his strikeouts and walks. However, the data set did not include home run totals, so I was forced to make do without H/IP instead.
Anyway, let’s move on to the meat and potatoes of my model: How these metrics are predictive of a pitcher’s big league performance. The graphic below plots some of my models’ coefficients across my entire spectrum of WAR thresholds. Basically, the further from zero a metric is, the more important it is. The metrics below zero — walks, and hits — are bad. A high strikeout rate is good, which is why it comes in above zero.

Strikeout rate clearly strays furthest from the x-axis, especially on the low end of the spectrum. This emphasizes how important it is that a college pitcher is able to miss bats. More often than not, it’s the high-strikeout college pitchers who go on to play in the big leagues. Walk rate and hit rate clearly both have a good amount of predictive value, but when it comes to forecasting college pitchers, strikeouts are king.
Finally, let’s look at a player’s class year. I normalized these coefficients to college freshmen. In other words, a freshman hitter would come in at “0” across the board. The chart below reflects the other classes’ distance from this group.

This chart looks a good deal like the one I produced for hitters on Friday. All else being equal, the earlier on a player is in his college career, the more impressive his performance is. Just as with minor league prospects, a standout season from a college prospect is more encouraging if he’s younger for his league. The drop-off between juniors and seniors isn’t nearly as stark as it was for hitters, where it was exceedingly rare for a senior sign to earn more than a few WAR. Still, it’s pretty clear that college pitchers who stick around for their senior seasons rarely make much of an impact in the big leagues.
Now that I’ve finished talking through the inner workings of my model, I’m finally ready apply all of my math to this year’s crop of draft-eligible college pitchers. Below, you’ll find the KATOH projections for the college hitters who are included in Kiley McDaniel’s handy (and sortable!) draft board. In a perfect world, I would have generated predictions for all current college players. However, Chris Long’s database does not include 2015 stats, and the data available from the team and conference websites does not include all of the data I’d need in a readable format. Nonetheless, I plan to gather all of the necessary data for the hitters taken in the first several rounds of the draft, and have forecasts for these players next week. In the mean time, let’s scout some stat lines!
KATOH 2015 College Pitcher Projections |
---|
WAR | |||||||||||||
---|---|---|---|---|---|---|---|---|---|---|---|---|---|
Kiley Rank | Player | MLB | >1 | >2 | >3 | >4 | >5 | >6 | >7 | >8 | >9 | >10 | Thru Age 28 |
5 | Carson Fulmer | 52% | 29% | 15% | 10% | 8% | 7% | 6% | 4% | 3% | 2% | 2% | 1.2 |
6 | Tyler Jay | 43% | 20% | 16% | 12% | 4% | 3% | 2% | 1% | 1% | 1% | 1% | 0.8 |
7 | Dillon Tate | 56% | 28% | 24% | 15% | 11% | 8% | 8% | 7% | 6% | 1% | 1% | 1.4 |
13 | Jon Harris* | 17% | 2% | 2% | 1% | 1% | 1% | 1% | 1% | 1% | 1% | 1% | 0.2 |
19 | Kyle Funkhouser | 17% | 4% | 2% | 1% | 1% | 1% | 0% | 0% | 0% | 0% | 0% | 0.2 |
22 | James Kaprielian | 56% | 35% | 30% | 23% | 20% | 18% | 17% | 16% | 14% | 14% | 14% | 2.9 |
23 | Walker Buehler | 20% | 9% | 3% | 2% | 2% | 2% | 1% | 1% | 1% | 1% | 1% | 0.4 |
29 | Nate Kirby | 33% | 9% | 6% | 2% | 2% | 2% | 0% | 0% | 0% | 0% | 0% | 0.4 |
32 | Cody Ponce* | 14% | 2% | 1% | 1% | 1% | 1% | 1% | 1% | 0% | 0% | 0% | 0.2 |
36 | Alex Young | 38% | 16% | 10% | 6% | 4% | 4% | 4% | 3% | 2% | 2% | 1% | 0.8 |
52 | Thomas Eshelman | 59% | 28% | 24% | 15% | 11% | 9% | 8% | 7% | 5% | 1% | 1% | 1.5 |
56 | Kyle Cody | 21% | 10% | 4% | 3% | 2% | 2% | 1% | 1% | 1% | 1% | 1% | 0.4 |
59 | Riley Ferrell | 76% | 48% | 37% | 24% | 16% | 14% | 15% | 14% | 8% | 7% | 7% | 2.6 |
61 | Andrew Suarez | 20% | 4% | 3% | 1% | 1% | 1% | 0% | 0% | 0% | 0% | 0% | 0.2 |
74 | Jeff Degano* | 23% | 3% | 2% | 2% | 1% | 1% | 1% | 1% | 1% | 1% | 1% | 0.3 |
79 | Josh Staumont* | 28% | 5% | 4% | 3% | 2% | 2% | 2% | 2% | 2% | 1% | 1% | 0.4 |
80 | David Hill* | 17% | 2% | 2% | 1% | 1% | 1% | 1% | 1% | 1% | 1% | 1% | 0.2 |
*Projected as though they pitched in the Atlantic Sun Conference
A few of the pitchers listed above — Jon Harris, Cody Ponce, Jeff Degano, Josh Staumont and David Hill — pitched in lower-tier conferences that did not make it into my regression model. For this reason, I treated them as if they had pitched in the Atlantic Sun Conference. Of the conferences built into my analysis, this is the one that dings pitchers the hardest across most of my WAR thresholds. I’m not sure if this is a fair treatment, to be honest, but I thought it was better to put out a possibly-flawed projection than to leave these guys off completely. Also, I excluded Michael Matuela, who didn’t pitch this year due to Tommy John Surgery.
The first thing you probably noticed was that these projections seem a little low. Not only are they much lower than the ones we saw for hitters on Monday, but they just feel low in general. Going by the table above, every college pitcher in the draft can be expected to produce less than three WAR by age 28. That’s pretty far-fetched.
For this reason, I’d recommend you pay less attention to the actual number in the far right column, and instead focus on how these pitchers rank relative to one another. Use this data to answer the question: “Will this pitcher be better than that pitcher?,” rather than “How good will this pitcher be?”
There’s a reason these projections are so low: It’s not easy to project college pitchers using only their stats. Some pitchers have very good stuff that doesn’t show up in the numbers. Some will inevitably get hurt. These statistical models use a pitcher’s statistics to learn as much as they can about a pitcher, but there’s still plenty that’s not accounted for. And for those unaccounted for areas, KATOH assumes a player’s just another member of the crowd. In college baseball, another face in the crowd is rarely a big leaguer.
Keep in mind that the pitchers I’m analyzing here are kids in their late teens or early twenties, many of whom are just starting to learn how to be “pitchers” rather than “throwers”. Plus, the competition they’re facing pales in comparison to the types of hitters they’ll see in the majors. Many college hitters aren’t even good enough to play minor league ball, and will be working white-collar jobs in a matter of months. This diluted competition surely muddies the calculus a bit.
Furthermore, there are pitchers who dominate in college, but whose stuff isn’t quite good enough to fool more advanced hitters. By that same token, there are also guys who have excellent stuff, but are still learning how to pitch. Pitchers of this ilk might put up middling numbers in college, but could be just an adjustment away from having everything click. Stats are only one piece of the puzzle.
Nonetheless, college pitching stats certainly aren’t meaningless, and as I showed above, some are more meaningful than others. Just looking at a pitchers stats allows you to make a decent guess as to how good he’ll ultimately become. And the data I’ve gathered implies that high-strikeout pitchers — such as TCU’s closer Riley Ferrell — are the best bets to turn into productive big leaguers.
Can you run this for last year’s draft class? I’d like to see the collective/average WAR difference relative to this year’s class. While still many years premature, I’d also like to do a bit of hindsight model evaluation.
Given the relatively high-injury rate of college pitchers who generate strike outs vs college hitters, would you consider the Katonah forecasts for college pitchers less predictive than the same for college hitters?
Yes definitely. Hitters are always more predictable than pitchers.
Assuming it’s fairly simple to do, can you run the numbers now on next year’s draft-eligible players?
I guess you were not able to do a projection for #19 Bickford because he pitched for a community college. Is it worth using Atlantic Sun as a proxy for him, or just too huge a talent difference to try?