Projecting Hanson
Note: I started this study before the season began and planned on submitting it then (took a lot longer than I thought obviously) so it’s only going to be using the information available prior to 2010. Later in the season I may revisit the topic incorporating the info gleaned from Hanson’s first half this year.
A few weeks ago here at THT, John R. Mayne presented his excellent research on how a pitcher’s velocity influenced whether or not he beat his preseason projections. He hypothesized that the pitchers who threw harder would beat their projections more often than those throw threw softer.
To investigate, John looked at the 20 pitchers with the worst fastball velocity in 2006 and the 20 pitchers with the best fastball velocity in 2006 and compared their CHONE ERA projections for 2007 with their actual ERA in 2007. He did the same thing for pitcher’s going into 2008 and 2009 as well. He used the “score” system, basically marking whether or not the pitcher’s performance was better, roughly equal to, or worse than his projection. For soft throwers he found that CHONE projected a lower ERA than the pitcher actually had in 44 out of 60 cases. For the hard throwers he found that CHONE projected a lower ERA than the pitcher actually had in just 22 cases.
The coolest part of John’s study was not what he actually found out about the relationship between velocity and performance relative to projections, but rather the idea behind it. In the opening sentences he explained that the point of his study was to explore how scouting can be used in conjunction with stats to better project players going forward. He hypothesized that given two pitchers with equal prior statistics, the one who scouted better would be more likely to perform better in the future. That may seem obvious. However, quantifying that effect is the part that is so hard. As a certain Tom Tango has frequently said, the convergence of scouting and performance is the pinnacle of sabermetric analysis, and John’s work was one of the best attempts so far at reconciling the two.
Today, I’d like to take John’s ideas a bit further. In his article, he only looked at the effects of fastball velocity, and anyone who’s ever seen a baseball game knows that is only one factor in a pitcher’s success. Movement, location, pitch selection, deception, etc. are all very important aspects of a pitcher’s skill set as well, and with the help of Pitch f/x data, I plan on taking a look at how one might combine a pitcher’s stats and those aspects to project future performance.
A brief note on projections
Recently, Brian Cartwright introduced his revamped Oliver projections, which are available for nearly 7,000 players if you sign up for THT Forecasts. His system now joins a whole host of other projection systems out their on the internet, including PECOTA, CHONE, ZIPS, Steamer, and Marcels (apologies to the owners of those I forgot).
Marcels, affectionately named after Marcel the Monkey, is the simplest of the systems. In fact, its creator Tom Tango deems them “the minimum level of competence that you should expect from any forecaster.” In other words, if you can’t beat Marcels than you are probably the projection system version of the Royals. The methodology can be boiled down into three steps:
1) Take a weighted average of a player’s previous three seasons.
2) Add in a certain amount of league average performance, otherwise know as regression to the mean.
3) Apply simple aging factors.
While the other, more complex systems have attributes that make them special, they all draw from the same basic framework as Marcels. And, as Tango recently showed at his blog, it’s very hard to beat the monkey.
The reason for this is pretty simple. Given that “noise” in each player’s seasonal stats (by “noise” I mean factors outside of the player’s control ), it’s necessary to regress those stats to the mean. In other words, say a hitter bats .330 in 600 at bats one year. We know that there are very few hitters who actually have the ability to sustain that high of an average given that the league average is around .260, so we’d project his “true” batting average going forward as somewhere in between.
The problem with that is that all players aren’t equally likely to hit .330. If the guy who batted .330 hitter is Ichiro – a guy who makes contact with everything, has blazing speed, places the ball and is halfway down the first base line when he makes contact – there would be no reason to expect him to regress to league average. But since the projection systems don’t know anything about Ichiro’s skill set, they are forced to regress him back to league average. Accordingly, he beats his projections every year.
However, if a projection system did know all of those things about Ichiro, they would not regress his batting average to the league average, but rather to that of players with similar skill sets. Those players might be expected to bat around .300, and by regressing Ichiro’s batting average to that, instead of to .260, his projections would be much more accurate. Therefore, it follows that the next step in projection systems is to be able to establish a given player’s skill set and regress his stats to those of similar players. That is exactly what I will be attempting to do today with Tommy Hanson.
Meet Tommy Hanson
Hanson was snatched up by the Braves in the 22nd round of the 2005 draft. He rose quickly through the minor leagues and pitched out-of-this-world at times, with his most notable achievement being a 14-strikeout no-hitter in 2008. After dominating the Arizona Fall League (0.63 ERA in five starts!) and Triple-A in 2009, he was called up to pitch for the Braves and was simply outstanding once again, pitching 127.2 innings and a measly 2.89 ERA. Somehow, even despite a BWWAA-friendly 11-4 record and much hype from the World Wide Leader, he finished third in the Rookie of the Year voting.
His performance thus far in professional baseball has been pretty amazing. With a bunch of help from the excellent Brian Cartwright and his Oliver projection system I gathered Hanson’s total innings pitched (IP), earned run average (ERA) and his park and league adjusted component BaseRuns ERA for each year that he’s been playing pro baseball:
+------+------------+-------+------+-------+ | Year | Level | IP | ERA | bsERA | +------+------------+-------+------+-------+ | 2006 | R+ | 51.2 | 2.09 | 4.39 | | 2007 | A/A+ | 133 | 3.32 | 7.41 | | 2008 | A+/AA/Fall | 166.2 | 2.11 | 3.53 | | 2009 | AAA/MLB | 194 | 2.42 | 3.34 | +------+------------+-------+------+-------+
That last column, the bsERA, represents our best guess of what his ERA would have looked like if he were playing the major leagues and in a neutral ballpark for that season. So while his ERA in Rookie Ball was an excellent 2.09, if he were suddenly transported to the major leagues, it likely wouldn’t have looked so good. How that number is calculated is pretty complicated and deserves some further explanation, but basically I (Brian) took seven different components of pitching (strikeouts, walks, hit batters, singles, doubles, triples, home runs), adjusted them by park and league, and estimated ERA from that. For more information, check out the “References and Resources” section at the end of the article.
Going forward, Hanson looks like one of the better young pitchers in baseball. He has a history of success at every level and at just 24 years old in 2010 has plenty of room for improvement. Given his past performance and expected improvement due to aging, you’d expect him to be somewhere in the 3.50 – 4.00 ERA range going forward. Indeed, THT’s own forecasting system projects a 3.54 ERA next season and the aggregate projection of the five other systems featured at FanGraphs is for a 3.38 ERA.
Scouting Tommy Hanson
Hanson’s a big guy, 6-6 and 220 according to FanGraphs, and throws from a high three-quarter arm slot, if that makes sense. According to Pitch f/x, his average release point was about 6 inches closer to the third base bag and 4 inches higher than the average right handed pitcher in 2009. For more info on Hanson’s delivery and mechanics, check out Alex Eisenberg at Baseball Intellect, who is a pretty big fan of what Hanson has to offer.
Anyway, that delivery produces the following amounts of velocity and movement on his pitches:
+------+----------+-------+-------+ | Type | Velocity | H-Mov | V-Mov | +------+----------+-------+-------+ | FF | 92.2 | -6.1 | 9.9 | | SL | 82.8 | 3.4 | 1.7 | | CU | 75.0 | 7.2 | -7.2 | | CH | 82.9 | -5.5 | 9.1 | +------+----------+-------+-------+
These are the averages for Hanson in 2009. Horizontal and vertical movement (technically known as “spin deflection”) measure how many inches the pitch moves in comparison to a theoretical pitch thrown without spin. That’s why you get some counter-intuitive numbers like his four-seam fastball having more horizontal movement than his slider. However, as Dave Allen has shown, counter-intuitive doesn’t necessarily mean those values are incorrect.
Looking at Josh Kalk’s Pitch f/x summary of “the league average pitcher” here at THT (parts 1 and 2), I can make some general observations about his stuff. Both the velocity and movement of his four-seam fastballs and sliders are similar to league average, although he throws a bit harder. His change-up, on the other hand, has roughly average velocity but has about 4 inches more “rise” than average and 1 inch less horizontal movement. As you can see above, it closely mirrors the movement of his four-seam fastball. That isn’t ideal for a change-up, as you would probably like some more separation from the fastball, but he doesn’t throw the pitch very often. His curveball looks to be his best pitch stuff wise, as it is thrown over 2 MPH slower than average and has about 3 extra inches of movement both ways – a real hammer.
He throws the fastball most often, obviously, at about 55 percent of the time. He then goes to the slider about 25 percent of the time, the curveball about 15 percent of the time and the change-up 5 percent of the time. That’s a pretty typical distribution of pitches from a right-handed starter with his repertoire, although you wonder why he doesn’t throw the curveball more often with the amount of movement it gets. Against left-handed hitters he cuts down on the slider and throws more change-ups, which is pretty standard as well.
The next most prominent part of a pitcher’s makeup is how he locates his pitches. This is a littler harder to get a handle on using Pitch f/x, primarily because we don’t know the intended location, but only where the ball ended up. Furthermore, pitchers will change their intended pitch location drastically by count, batter type, previous pitch, etc., but when you try to group 1,900 pitches into a bazillion different subsets, the sample sizes are so small as to be meaningless. However, while flawed, looking at the aggregate location by pitch type still has some value.
To do this I split the strike zone into nine different zones as shown on the right and measured the percentage of each pitch type thrown in each zone. I then did the same for all pitches in my database thrown by a right-handed pitcher as a means for comparison. Using those zones, some things immediately jumped out at me. For one, Hanson pounded the strike zone with his slider, throwing over 55 percent pitches inside the strike zone and over 21 percent in the middle section. He located his fastball pretty well, throwing fewer pitches in the strike zone, but more on the corners than league average. He threw his change-up and curveball out of the strike zone significantly more than league average, but with the amount of movement his curve gets, that’s not such a bad thing. His change-up just doesn’t appear to be a very good pitch in terms of either stuff or location, but again, given that he only throws in 5 percent of the time, it’s not a big factor in his repertoire.
The full data for each pitch type thrown by Hanson and the league average numbers can be found here, and if you want to see the actual scatterplots by pitch type, click on the following thumbnails:
There is obviously a lot more to pitching than what I examined above; however, in my opinion, release point, pitch selection, “stuff” and location are the most important aspects of pitching, and breaking the data down any further wouldn’t likely produce much more useful information. The takeaway from this is that, at first glance, Hanson looked solid, but not great, in the scouting department last year, as least according to Pitch f/x. His stuff didn’t overwhelm, with the exception of the curve, and his location wasn’t anything special. He was above average for sure, but not quite at the level you’d expect from a guy with his hype and past performance.
Establishing the mean
From scouting Tommy Hanson through Pitch f/x data there are certainly some things we learned about the skill set he displayed in 2009. The tricky part is quantifying how that skill set translates into expected performance. There are a couple of possible ways to do this, but the one that makes the most sense to me is similarity scores. Basically, sim scores are a comparison of how closely two players are related when looking at a certain set of components. They were popularized by Bill James to compare players’ stats, and each player’s most similar players are displayed at Baseball Reference. In this case I used Pitch f/x data to compare Hanson to each other pitcher in the majors in 2009, incorporating release point, pitch selection, stuff and location. For more info on how the sim scores were calculated, check out the “References and Resources” section at the end of the article.
So without further ado, I present the top 10 most comparable pitchers for Hanson in 2009:
+------------------+-----------+ | Player | Sim score | +------------------+-----------+ | Scott Richmond | 0.90 | | Zack Greinke | 0.90 | | Ricky Nolasco | 0.89 | | Joba Chamberlain | 0.89 | | Matt Garza | 0.88 | | Takashi Saito | 0.88 | | Brett Tomko | 0.88 | | Kevin Gregg | 0.87 | | Seth McClung | 0.87 | | Sean Gallagher | 0.87 | +------------------+-----------+
The units for the similarity scores are the percentage of similar “area” shared by the two pitchers. A carbon copy of Hanson would have had a sim score of 1.0 last year, while guys like Tim Wakefield and Brian Shouse have around a .20 score and a completely dissimilar pitcher would have a score of 0. It’s kinda complicated how those numbers are actually derived, but I think that’s a pretty good summarization of it for now.
As you can see, while some of Hanson’s most similar pitchers were very good in 2009 (Greinke, Garza, Saito, Nolasco if you are an FIP fan), once you get more down the list there are some ugly comparables. Brett Tomko is a career journeyman who had a decent ERA, but ugly peripherals in 2009. Sean Gallagher is a guy with good stuff, but also a career 5.65 ERA in the majors. Seth McClung… is Seth McClung. That massive differences in production between some of the guys most similar to Hanson in my opinion speaks to the idea that baseball is a game of inches. Very little separates the best pitchers in the game from the worst once you get to the major league level, but those little differences can go a long way. Either that, or my sim scores algorithm just sucks. Let’s stick with the first explanation for now.
Anyway, translating those sim scores into an explicit number is a pretty simple process. With the help of Brian Cartwright once again, I gathered the 2009 park and league adjusted stats for each of Hanson’s top 50 most similar pitchers in 2009, including those who spent time in the minors. I then took a weighted average of each of the seven components used in the BaseRuns ERA formula in the “Meet Tommy Hanson” section of the article. Here is what I got, with the league average numbers displayed below for reference:
+----------+-------+------+------+-------+------+------+------+ | | K | BB | HBP | 1B | 2B | 3B | HR | +----------+-------+------+------+-------+------+------+------+ | Similars | 19.2% | 8.2% | 0.8% | 15.4% | 4.6% | 0.5% | 2.6% | | League | 18.0% | 8.3% | 0.8% | 15.4% | 4.7% | 0.5% | 2.7% | +----------+-------+------+------+-------+------+------+------+
The BaseRuns calculation yields an expected ERA of 4.11. However, since we really care most about Hanson going forward that line has to be adjusted for aging. To do that I ran my similarity scores for Hanson’s 2009 season against each pitcher in 2008. I then looked at all pitchers who were 24 in 2008 and 25 in 2009 and did a weighted average, by degree of similarity and plate appearances, of the difference of each of the seven components above from 2008 to 2009. The aging adjustment was somewhat powerful, taking away some walks and hits allowed, and producing a BaseRuns ERA of 3.85.
The fun stuff
Now if my similarity scores algorithm was perfect (it isn’t!) and encompassed all aspects of pitching (it doesn’t!) and my aging curve will perfectly model Hanson’s career trajectory (it won’t!), that 3.85 ERA would represent exactly how well Hanson should pitch in 2010 given his skill set displayed in 2009. However, because so there is so much potential room for error in the analysis conducted above, Hanson’s actual performance needs to be taken into account as well.
To do that I modeled what Hanson’s projection would look like if we only took his stats into account. This is somewhat of a tricky business because some of the stats above (like strikeout percentage) are more in the pitcher’s control than others (like triples percentage), so each stat has different amounts of predictive value. Furthermore, Hanson’s stats three years ago are obviously not as predictive as those from last year, so that needs to be taken into account as well. After some months of thought (not really though) I came across a simple but elegant solution to the problem. For all pitchers who faced at least 200 batters in 2006, 2007 and 2008, I ran separate multiple regressions for each of the seven components on those pitchers’ 2009 numbers. The coefficients for those regressions, with the y intercept set at 0, gave me the amount of weight to give to each of year.
I then took a weighted average of Hanson’s MLE’s over the past three seasons using those coefficients. When plugged into the BaseRuns ERA formula, I got an expected ERA of 3.67 for Hanson. The next step is to reconcile that number with his expected ERA when looking solely at his top Pitch f/x comparables in 2009. To do that I found the correlation of the weighted average of 2006, 2007 and 2008 for 2009 for each of the seven components. Strikeout rate was the most stable, with a .57 correlation, while triples rate was the least stable with a .09 correlation. That correlation allows us to figure out how much to regress each component to the mean, with the higher the correlation the less the amount of regression.
After regressing each component of Hanson’s past performance to the mean of his most similar pitchers and plugging those number through the BaseRuns formula, I get an expected ERA of 3.67 going forward for Hanson. You’ll notice that’s the exact same thing as his past performance numbers. The reason for this is that his singles rate on balls in play regressed heavily up to that of his comparables, while his home run rate regressed heavily down to that of his comparables. The two canceled each other out.
In the case of Hanson, this extremely complicated analysis yields some pretty boring results. Essentially, Hanson projects to pitch just as well as he always has because his past performance and the performance of players with similar skill sets are very similar. What is interesting is the distribution of possible numbers for Hanson in 2010. Because estimating his most similar players is an inexact science, I used the average of Hanson’s most similar players to establish his mean. However, it’s possible that Hanson really has the same skill set as Zack Greinke or Matt Garza, in which case his projected ERA would be much lower than the mean. It’s also possible that Hanson is really a guy like Brett Tomko or Sean Gallagher.
So I ran the projection algorithm again, this time, instead of regressing Hanson’s stats to the average statline of his most similar players, I regressed Hanson’s stats to each individual pitcher among his top 50 most similar pitchers. When plotted out in a histogram you can see the expected range in Hanson’s performance for 2010:
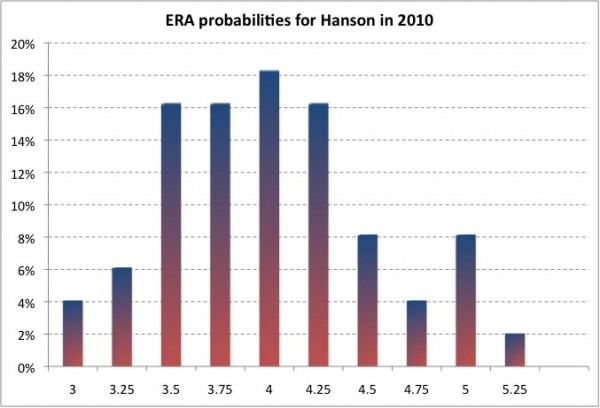
Using those numbers, we can estimate that Hanson has roughly a 10 percent chance at a breakout (less than a 3.50 ERA) and 14 percent chance at a flop (greater than a 4.75 ERA). His most likely performance is for around a 4.00 ERA while a weighted mean yields a 3.67 ERA as I said above.
Conclusion
So that’s about it. Despite the rather… predictive projection for Hanson, I feel that the idea of incorporating Pitch f/x into objective pitcher projections is a worthwhile task and I hope I was able to outline the start of a framework for doing so. There are certainly many, many problems with the specific approach I took (some known and some unknown to me) and I hope those can be fleshed out and improved upon by the many fine readers of THT.
References & Resources
Pitch f/x data provided by MLBAM and Sportsvision, pitch classification for Hanson hand done by myself for best accuracy.
Here are the year by year regression results for the seven components:
+------+------+------+------+ | | 2006 | 2007 | 2008 | +------+------+------+------+ | K | 0.15 | 0.23 | 0.62 | | BB | 0.21 | 0.25 | 0.51 | | HBP | 0.20 | 0.29 | 0.42 | | 1B | 0.28 | 0.34 | 0.36 | | 2B | 0.20 | 0.33 | 0.43 | | 3B | 0.24 | 0.27 | 0.35 | | HR | 0.20 | 0.30 | 0.35 | +------+------+------+------+
These are the coefficients with the y-intercept set at 0, so to predict strikeout rate in 2009, you would take 62 percent of a players strikeout percentage in 2008, 23 percent of 2007 and 15 percent of 2006. As you can see, some stats like singles and triples rate are much more even across multiple years, implying that a larger sample is needed before the numbers are very predictive. In other words a player’s strikeout rate in 2008 is much more likely to be predictive for 2009 than his singles rate.
The BaseRuns ERA formula I used to convert the component stats into an expected ERA is:
A = H + W - HR + HB B = (1.4*TB - .6*H - 3* HR + .1*(W + HB))*.94 C = .975*(PA - H - W - HB) D = HR BaseRuns = A*B/(B + C) + D BaseRuns ERA = BsR*9/IP *.92
Graciously provided by Patriot. I used Brian Carwright’s MLE numbers for each pitcher since 1998. Those MLE’s are park and league adjusted and explained in more detail here.
The similarity scores were created by grouping pitchers into 56 different components: average horiziontal and vertical release point, average velocity for each pitch, average horizontal and vertical movement for each pitch and percentage of each pitch type out of total pitches thrown in each of the five zones I used in the “Scouting Tommy Hanson” section of the article. To get each category on the same units (1 MPH in velocity is not the same as 1 inch of movement), I figured out the standard deviation for each across all pitchers in 2009. From there it was simple to compare two pitchers:
| Hanson category A - Pitcher 2 category A | / standard deviation category A + | Hanson category B - Pitcher 2 category B | / standard deviation category B + ... | Hanson category BD - pitcher 2 category BD | / standard deviation category BD
I did that for all pitchers then averaged out the results. So, for example, Scott Richmond and Tommy Hanson last year were within .24 standard deviations from each other on average. To convert that to percentage of shared area, I divided the difference by 2 to get a quasi Z-Score and performed some integral calculus to find the expected area of a bell curve taken up by the difference in standard deviations between two pitchers. I then subtracted that number from one, and voila! Average percentage of similar area shared by each group of two pitchers across 56 different categories. There is a little bit of fudging involved, but nothing too crazy I don’t think. Any statisticians reading this should feel free to say where I screwed up.
Special thanks to Patriot, Tom Tango, Brian Carwright, Paul Singman and David Gassko for help with this article.
Not a statistician, but rather just a philosopher here. Read the book “How we decide” and consider this: Often times, the more variables we consider the worse we do in predictions.
There are many reasons for this, but have you compared the results of your method against other simpler ones? I understand you are just throwing this out there, but I would contend that breaking things down to such a level makes analysis less meaningful, not more. Keep this possibility in mind as you continue to search for the holy grail of predictive systems. Accept there are going to be some things that you cannot know, and cannot predict, before you start. Just because the math works does not mean it is reflecting reality.
Dan – the sim scored used was the simplest possible one I could think of. I didn’t break down by better handedness or count or more precise location vectors.
I only used velocity, movement, release point and location in the 5 zones to make the scores. If you have any suggestions to make that simpler, please let me know.
Nick, you may be on to something here, but you are using Velocity, Movement, Release Point, and 5 zones of location, which equate to hundreds of thousands of possible outcomes. Such a scheme is extremely complex without accounting for handedness, count, or any other obvious additions.
My point is such a complicated model is very likely not going to be much better than taking last year’s FIP and accounting for aging. Indeed, it might be much worse. You are assuming that more factors with automatically improve your models, when experience shows this is not likely.
I have no suggestions for making your model simpler, I am just cautioning you to consider the results and compare to other, simpler models for efficacy as you attempt your project.
Fantastic article. I have just recently started to get into sabermetrics and had a couple of questions/comments.
1) Have systems like neural networks been used to predict player statistics? I know that they can be good predictive tools, but have an upper bound to accuracy due to noise inherent in the training set, which you alluded to when talking about Marcel. It might be an interesting route to explore.
2) For your regression, was everything (singles, doubles, triples, HR, etc) normalized to the ballpark? I may have just missed that.
3) It would be interesting to try to do predictions on 2009 player statistics. That way you could concretely test with “experimental” verification how the players performed. I work in computational biology and hear (unfortunately) way too often that our predictions need experimental verification.
I just hope the guy stays healthy. ANY degree of injury probably blows up the data
Nick, you may be right, I may be wrong, but my only point is that the more stuff you throw into a model, the less accurate it generally gets, not more. Espicially when you are dealing with human performance rather than something more objective. But good luck anyway.
Great work, Nick. The notion of regressing a player’s historical stats to the mean of players with similar skill sets and physical attributes (and whatever else contributes to performance) is an important one. I recently started doing that with my pitcher projections with respect to pitcher velocity, much like the Mayne article you cite above (that is essentially the reason why pitchers with higher velocities outperform their projections and vice versa – they are both being regressed to the same mean, which is incorrect – pitchers with higher FB velocities have better average numbers), and I feel like I have much better projections.
You are taking it one step further which is excellent. Obviously the exact methodology needs some tweaking, but the concept is great and necessary.
BTW, when you compute the means of the pitchers on the sim list, you MUST adjust for whether they are starters or relievers, even though they have they all have the same characteristics. The reason is that a reliever with exactly the same stuff will still perform better (than a starter with the same stuff) because he faces the order only once (generally) and pitches when it is a little colder (generally).
Nick: have you considered converting the pitch attributes (velocity, movement, and location) into average run values? That is, what is the average run value for sliders when thrown at Hanson’s velocity, with his movement, and his distribution of locations? Regressing to that might be as good or better than using comparable pitchers, and strikes me as much easier.
Great work…..
Guy, I thought about that, but with similarity scores I hoped to be able to capture the effects of the interrelations between pitches. For example Hanson’s changeup might be a below average pitch based off it’s location/velocity/movement, but when you add it to the rest of his plus repertoire and the fact that he throws it very infrequently and only to lefties, it may in fact have a positive run expectation.
There is obviously a tradeoff as run values are a lot easier to manipulate as you say, but I think using sim scores may incorporate more information. Thanks for the comment.
Nick you jinxed Hanson. lolz.
Hey I said it was possible he could turn out like Brett Tomko…
Dan – I’m confused about what you are trying to say. The whole point of this article was to combine stats and scouting data to project pitchers going forward. You may be right that a simple Marcel’s system would be better, but the whole point of this was to try and establish a more complex system. I feel that the idea of regressing stats to the mean of similar pitchers is a good concept and one that if executed right could be an improvement over the simpler projection systems.
Aaron – Thanks. I’ll try to address your points:
1) I’m not aware of Neural Nets being used for projections, and although I’m slightly familiar with those methods I have nowhere near the skills to be able to competently apply something like that.
2) I used park and league adjusted MLE’s for the entire article, so everything was adjusted for context.
3) I agree that testing how predictive this method is is the ultimate test, and when I figure out how to automate the process, that’s exactly what I’ll do. For now, I am just trying to put the methodology out there and get some feedback.
MGL – Thanks for the comments. I’m not surprised that you’ve started doing something like this (I think Rally also mentioned he was going to start it). That’s a good point about adjusting for relievers. I went through that debate in my head, but I decided that, like you said, there shouldn’t be much of a difference between a starter and a reliever if both had similar stuff/location/selection. I didn’t think that one other difference might be the context. How should I adjust for relievers? Should I just eliminate them from my data set or apply some generic adjustments?
Nick,
This is the best dabermetric article I’ve read this year. Not much for the outcome, but the methods are incredibly exciting in their potential. Love that you not only give us pitchfx-relevant projections but also distributions for those projections. This is groundbreaking stuff. Looking forward to seeing where you and others take this.
-j
Thanks a lot Justin! I do pride myself on my dabermetrics.
Damn iPhone not autocorrecting sabermetrics…
Great job Nick. The beauty of this is breaking down the pitcher the way a scout does, and then putting numbers to it! Simpler is not always better than “as simple as possible”. Other systems are good, but this way serves to a wider purpose. Beyond MLB level, could help to increase accuracy assessing minor leaguers and guys coming out college/HS. Breaking down Tommy is a good start to extend this… Imagine data of the next victim: Strasburg plotted like this… Holy Grail on the way!
Not that this means much, but so far Hanson has a 3.78 ERA this year compared to my projection of a 3.67 ERA